NO.189 Advancing Visual Computing in Materials Science
May 13 - 17, 2024 (Check-in: May 12, 2024 )
Organizers
- Christoph Heinzl
- University of Passau, Germany
- Renata Georgia Raidou
- TU Wien, Austria
- Kristi Potter
- National Renewable Energy Laboratory (NREL), USA
- Yuriko Takeshima
- Tokyo University of Technology, Japan
- Mike Kirby
- University of Utah, USA
- Guillermo Requena
- DLR - RWTH Aachen University, Germany
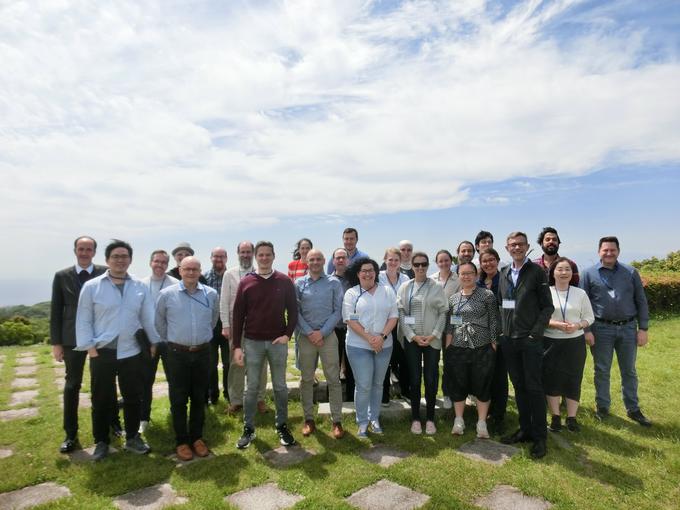
Overview
1. Overview
Materials science has a signifcant impact on society and its quality of life-for example, through the development of safer, healthier, more durable, more economic, environmentally friendly and sustainable materials. Visual computing in materials science integrates computer science disciplines from image processing, visualization, computer graphics, pattern recognition, computer vision, virtual and augmented reality, machine learning, to human-computer interaction, in order to support the acquisition, analysis, and synthesis of (visual) materials science data with computer resources. Therefore, visual computing may provide fundamentally new insights into materials science problems by facilitating the understanding, discovery, design, and usage of complex material systems (1).
We consider this seminar as a follow-up of the Dagstuhl seminar (n.19151), held in April 2019 (2). Since then, the field has kept evolving and many novel challenges have emerged, with regard to more tra-ditional topics in visual computing, such as topology analysis or image processing and analysis, to recently emerging topics, such as uncertainty and ensemble analysis, and to the integration of new research dis-ciplines and exploratory technologies, such machine learning and immersive analytics. The 2019 seminar aimed at setting the fundamentals of the field and stimulating co-operations with domain experts. With the current seminar, we target to strengthen and extend the collaboration between the domains of visual computing and materials science (and across visual computing disciplines), by foreseeing challenges and identifying novel directions of interdisciplinary work. We have included six organizers: Christoph Heinzl (Visual Computing in Materials Science expert), Mike Kirby (Scientific Computing and Visualization expert), and Guillermo Requena (Materials Science expert) were co-organizing the previous Dagstuhl seminar. Kristi Potter (Uncertainty Visualization expert) was a participant at the previous Dagstuhl seminar. Yuriko Takeshima (Topology-based Visualization expert) and Renata Raidou (Visual Analytics expert) are new additions to the team. The choice of organizers reects the balance between traditional and recently emerging research topics.
During the proposed 5-day seminar, we aim to bring visual computing and visualization experts from academia, research centers and industry together with domain experts, to uncover the overlaps of visual computing and materials science and to discover yet-unsolved challenges, on which we can collaborate to achieve a higher societal impact. We see this as a great opportunity to cover a range of relevant and contemporary topics, and to identify the most significant directions for future work. By organizing the seminar at the Shonan Village Center, we aim at bringing together top-notch researchers from the entire world, and especially at bridging new collaborations with Asian institutions.
2. Aims of the Seminar
In our seminar, our goal is to bring together researchers working in close proximity to the interdisciplinary domain of visual computing in materials science, in order to identify current, unsolved challenges and to discuss opportunities for future collaborations. This cannot be done easily during conferences, as there are no dedicated venues, where both visual computing and material science experts participate, and can discuss in an organized and structured manner. Topic-wise, our seminar aims at covering the most relevant trends within visual computing and linking them to current and future challenges in materials science. We, therefore, aim to discuss topics that address analytical processes through their entire spectrum|from exploration to decision making. These topics span from traditional directions, such as topology analysis, to recently emerging directions, such as uncertainty and ensemble analysis, and to new technologies, such machine learning and immersive analytics.
We expect that our seminar will contribute to new interesting paths of research and will significantly advance the domain of visual computing in materials science in many ways, which is simply impossible without this seminar. Despite the specifc objectives of the application domain, we anticipate that our joint effort might be useful and applicable to other domains of interdisciplinary work.
3. Topics of the Seminar
We have identified three main directions, which have not been tackled in depth or at all at the previous Dagstuhl seminar and we would like to address them at our Shonan seminar. These are: Topology visualization, Integrated visual analysis and Interpretability and decision making. We have categorized these directions (and their respective topics) based on two dimensions: traditional research vs. new trends and exploration vs. decision making. We summarize our categorization in the figure below.
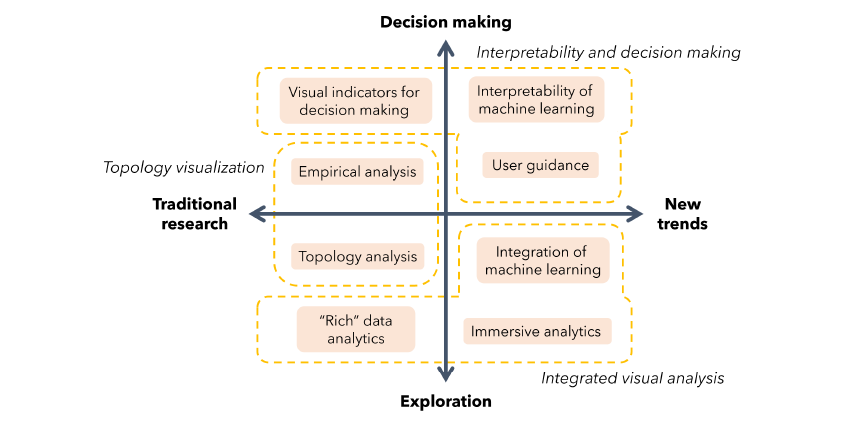
Topology-based visualization for materials science: To effectively visualize large-scale and complex datasets in aterials science, a data-centric approach based on the structural feature of the target dataset is indispensable. In particular, topology is an effective structure for analysis in scientific applications. In this seminar, we would like to discuss two main topics from the theoretical and practical perspectives in topology-based visualization:
-
Topological analysis of materials: We target a discussion on the signi cance of the topology of inherent features, such as complex internal structures (e.g., pore or crack network analysis, permeability analysis) in materials science. We mainly consider the physical meaning of the topology in materials science, and also discuss the seeds and needs for topology-based visualization.
-
Empirical analysis of persistency: The topological analysis of practical datasets extracts many critical points, as the target dataset involves small amplitude noise in some cases. Such minor critical points might be hiding an important global structure within the dataset, and we should remove them appropriately. We will discuss strategies to evaluate their importance and methods to reduce them effectively.
Integrated visual analysis is of core interest in materials science. Aside from the data analysis and data exploration aspect, quantitative data visualization is also of high relevance and highly challenging in the materials science domain, e.g., for precise modeling and simulation of material systems. Features of interest need to be extracted in the data over spatial, temporal or even higher dimensional data domains and are crucial to refine material simulations, as well as predicting material properties. In this seminar, we target to discuss three novel streams coming in, which show high potential in this domain:
- "Rich" data analytics: Visual analytics has become particularly popular within the domain of materials science. We target a discussion on the best practices and challenges of designing, developing and employing scalable solutions for the quanti cation, exploration and analysis of "rich" materials science data, i.e., multi-dimensional, multi-faceted, complex, and possibly timevarying and/or multi-modal data. Within this discussion, we will incorporate topics from the domains of ensemble and comparative visualization.
- Integration of machine learning: Deep Convolutional Neural Networks (CNNs) and corresponding classi ers have been able to identify and classify features with very high probabilities in various application areas. Current approaches have successfully trained and applied Deep CNNs to segmentation, for feature extraction or the reconstruction of XCT data. We, thus, strive for a discussion on how machine learning concepts can be employed in visual computing in materials science, facilitating knowledge transfer between materials and avoiding errors in training.
-
Immersive analytics in materials science: We target a discussion of how immersive analytics (AR/VR) can substantially help data analysis and exploration in materials science or if it rather hinders it because of novel interaction concepts, complex metrics for complex data and thus steeper learning curves. Furthermore, it needs to be clarified if current visual metaphors are enough or if tailored representations are required.
Interpretability and decision making: The complexity of materials systems, as well as the complexity of state-of-the-art visual analytic tools, features the huge potential to compound challenges in understanding results and making decisions. Specifically, advances in machine learning (ML) are quickly allowing for approximations of large-scale simulations, as well as automated analytics and decision making. However, in many cases a human-in-the loop approach will be required to ensure safe and appropriate outcomes. New techniques will be required to facilitate understanding of automated tools, as well as uncertainty arising in these tools. We will discuss three topics towards this direction:
- Interpretability of machine learning methods: ML is often used for dimension and complexity reduction, or to approximate models that can quickly provide insights on the data. However, ML is only as good as its training. In the materials domain, without appropriate and careful training, a machine may generate models that, e.g., use infeasible or ill-advised parameter settings-issues that may easily be avoided through the intervention by a knowledgeable, human, expert. To this end, these techniques must be accompanied by visual tools to support the interpretability of what the methods are doing, as well as by new interaction techniques.
- Visual indicators for decision making will also be required. This includes visualization methods for input parameter spaces and output quantities of interest. Furthermore, understanding the uncertainty within these methods is paramount for decision making. Without knowledge of where and why a model might fail, any decision made using these tools may be faulty. While the in uence of parameters and resulting uncertainties are topics in visual computing in general, there are likely to be speci c challenges in the material domain, which should be identified.
- Knowledge-driven and guided analysis: We target a discussion on how visual analytics for the materials science can benefit from new concepts, such as guidance and knowledge-driven exploration--especially, in conjunction with the integrated visual analysis of "rich" data mentioned above. As the complexity and richness of the data increases, these topics will become indispensable components of visual analytics solutions aiming at actively resolve a knowledge gap encountered by users, during their analytical processes.
Overarching connection between topics: The aforementioned discussion directions are not completely detached from each other. For example, the topology-related topics are closely related to the "rich" data analytics, and the integration of ML and immersive analytics are linked to the interpretability topics. This connection is intended, as we would like to create a linkage between the different discussion groups and to discuss future challenges of the domain in a more holistic manner.
4. Expected Outcome
The expected outcome of the seminar will be a cumulative position paper that will communicate to the broader visual computing and materials science community the outcomes of our discussions during the seminar. We expect that this seminar should also trigger a compilation of publications, related to all topics addressed during the event. Especially, the future challenges will be summarized and published to the broader visualization community--either with a short communication, or with a tutorial or panel discussion at one of our venues, such as IEEE VIS. The same should be done also with regard to the domain of materials science in conferences such as WCNDT, or EuroMat.
References:
(1)Heinzl, C. and Stappen, S. Visual Computing in Materials Science, Computer Graphics Forum (36:3), 2017.
(2)Dagstuhl-Seminar 19151 Visual Computing in Materials Sciences https://www.dagstuhl.de/19151
Cite As
Christoph Heinzl, Renata Georgia Raidou, Kristi Potter, Yuriko Takeshima, Mike Kirby, Guillermo Requena. "Advancing Visual Computing in Materials Sciences" (Shonan Seminar 189). Shonan Seminar Report 189 pp. 1-63, Shonan Village Center, NII National Institute of Informatics, (05/2024), /seminars/189/