NO.140 Optimization Methods in Geometric Vision
January 28 - 31, 2019 (Check-in: January 27, 2019 )
Organizers
- Tat-Jun Chin
- The University of Adelaide, Australia
- Anders Eriksson
- Queensland University of Technology, Australia
- Yasuyuki Matsushita
- Osaka University, Japan
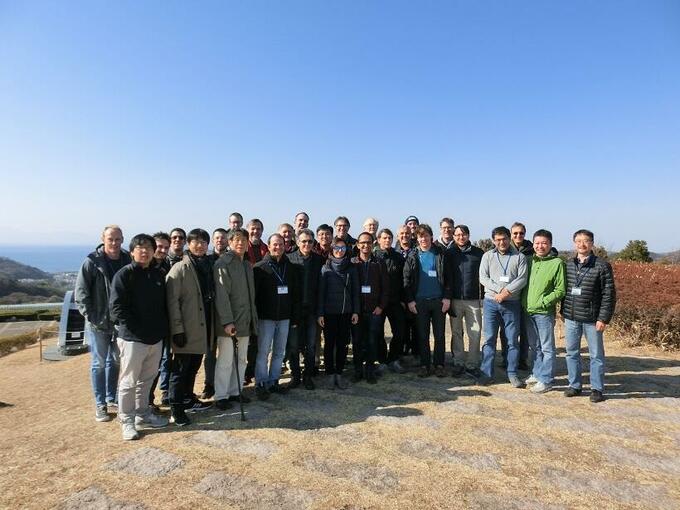
Overview
Background
Computer vision is concerned with inferring properties of the world from observations in the form of visual images. Such inverse problems typically take shape as optimization problems, that aim to find the best explanation, for the complex visual phenomenon that gave rise to a set of noisy and incomplete visual measurements. For computer vision applications to be successful, the underlying optimization problems must be supported by efficient and dependable solution methods.
The proposed meeting focuses on a broad subclass of computer vision problems called geometric vision problems. Roughly, these are problems that exploit fundamental geometrical constraints arising from the image formation process or physical properties of the scene (e.g., lighting conditions, characteristics of motions), to extract information of the scene (e.g., depth, 3D shape, camera trajectory, object identities) from the given visual data. Example geometric vision problems include structure-from-motion (SfM), simultaneous localization and mapping (SLAM), pose averaging [1], photometric stereo [2], and motion segmentation [3]. Methods for solving geometric vision problems underpin many useful applications, such as 3D reconstruction, robot navigation, object recognition/tracking, and computational photography [4].
Geometric vision is replete with hard optimization problems. By “hard”, we mean that the time needed to solve the optimization problems grows quickly with the size of the input data. Take, for example, the task of robustly estimating the planar perspective transformation (a.k.a. homography) from outlier-contaminated point correspondences between two images. Due to the inherent intractability of robust homography estimation [5], practitioners often rely on simple randomized heuristics to find rough approximate solutions, which neither guarantee optimality nor provide bounds on the approximation error.
The computational difficulty of geometric vision problems is also often compounded by the extremely large size of the input. Take, for example, the task of bundle adjustment, i.e., calculate 3D points and camera poses that are consistent with a set of images of a scene. In the age of big data, the input image set is often obtained by “scraping” Internet photo collections, or by conducting long-term surveillance of a scene using a robot. Such input sizes easily overwhelm traditional computing architectures, and distributed or parallel versions of bundle adjustment must be used [6].
Technical themes
The overall theme for the proposed meeting is recent theoretical and algorithmic advances on optimization
problems in geometric vision. More specific themes include:
- Solvability and approximability of geometric vision problems, e.g., [5,7];
- Duality in geometric vision problems, e.g., [8,9,10];
- Global optimization algorithms for geometric vision, e.g., [5,10];
- Approximate algorithms including randomized methods, e.g., [11,12];
- Distributed algorithms for geometric vision problems, e.g., [6]; and
- Incremental algorithms for online geometric optimization, e.g., [7,12].
Apart from discussing recent progress in geometric optimization through the above themes, we also aim to
chart future research directions and novel application areas. For example:
- The role of machine learning in geometric optimization;
- Geometric optimization on constrained computing platforms (e.g., smartphones, sensor networks);
- Geometric optimization for novel imaging devices (e.g., RGBD cameras, light field cameras); and
- Geometric vision problems from new industries (e.g., self-driving cars, UAVs).
Following the spirit of Shonan Meetings, we will also consider other related topics, based on the interest of
the attendees and the trajectory of the discussions.
Aims and intended impact
The main aim of the proposed meeting is to make progress towards answering some of the more fundamental questions in geometric vision:
- Why are certain geometric vision problems hard?
- What properties differentiate geometric vision problems from other optimisation problems?
- Are there “hard” problems that are actually easy?
- What are the good ways to construct approximate solutions for geometric vision problems?
It is hoped that the meeting will contribute towards promoting the value of basic algorithmic research in computer vision, especially in the area of geometric optimization.
From a more practical standpoint, and as alluded to above, geometric vision problems underpin some of the most important capabilities (e.g., 3D sensing and navigation, object recognition and tracking) that support intelligent machines. Therefore, the outcomes of the proposed meeting could have significant impact on some of the most important technological developments, such as self-driving cars, intelligent domestic robots, and smart manufacturing—developments that are already driving the economic growth in developed and developing countries alike.
Structure
The proposed meeting will follow the standard 4-day seminar timetable, which includes four mornings and two afternoons of technical discussions, and an excursion on the afternoon of the third day.
To enable the sharing of accumulated experience from broader areas (computer vision, robotics, control), we are pleased to have the following highlight speakers as special guests to our meeting:
- Fredrik Kahl, Chalmers University
- Frank Dellaert, Georgia Tech
- Richard Hartley, Australian National University
References
[1] R. Hartley, K. Aftab and J. Trumpf. L1 rotation averaging using the Weiszfeld algorithm. In IEEE Conference on Computer Vision and Pattern Recognition (CVPR) 2011.
[2] J. Park, S. N. Sinha, Y. Matsushita, Y.-W. Tai, and I. S. Kweon. Robust multiview photometric stereo using planar mesh parameterization. IEEE Trans. on Pattern Analysis and Machine Intelligence (PAMI), 2016.
[3] T.-J. Chin, H. Wang and D. Suter. The ordered residual kernel for robust motion subspace clustering. In Advances on Neural Information Processing Systems (NIPS) 2009.
[4] Y. Matsushita, E. Ofek, W. Ge, X. Tang, and H.-Y. Shum. Full-frame video stabilization with motion inpainting. IEEE Trans. on PAMI, 28(7), pp. 1150-1163, July, 2006.
[5] T.-J. Chin and D. Suter. The maximum consensus problem: recent algorithmic advances Synthesis Lectures on Computer Vision (Eds. Gerard Medioni and Sven Dickinson). Morgan & Claypool Publishers, Feb 2017.
[6] A. Eriksson, J. Bastian, T.-J. Chin and M. Isaksson. A consensus-based framework for distributed bundle adjustment. In IEEE CVPR 2016.
[7] Q. Zhang and T.-J. Chin. Coresets for triangulation. IEEE Trans. on PAMI, accepted on 4/9/2017.
[8] K. Wilson, D. Bindel, and N. Snavely. When is rotations averaging hard? In European Conference on Computer Vision (ECCV) 2016.
[9] L. Carlone, G. Calafiore, C. Tommolillo, F. Dellaert. Planar pose graph optimization: duality, optimal solutions, and verification. IEEE Trans. on Robotics, 32(3):545-565, 2016.
[10] A. Eriksson, C. Olsson, F. Kahl, O. Enqvist, T.-J. Chin. Why rotation averaging is easy. Arxiv, 2017.
[11] T.-H. Oh, Y. Matsushita, Y.-W. Tai, and I. S. Kweon. Fast randomized singular value thresholding for nuclear norm minimization. In IEEE CVPR 2015.
[12] H. M. Le, T.-J. Chin and D. Suter. An exact penalty method for locally convergent maximum consensus. In IEEE CVPR 2017.