NO.211 Theory and Algorithms in Graph Rigidity and Algebraic Statistics
September 2 - 6, 2024 (Check-in: September 1, 2024 )
Organizers
- Fatemeh Mohammadi
- KU Leuven, Belgium
- Bernd Schulze
- Lancaster University, UK
- Meera Sitharam
- University of Florida, USA
- Shin-Ichi Tanigawa
- University of Tokyo, Japan
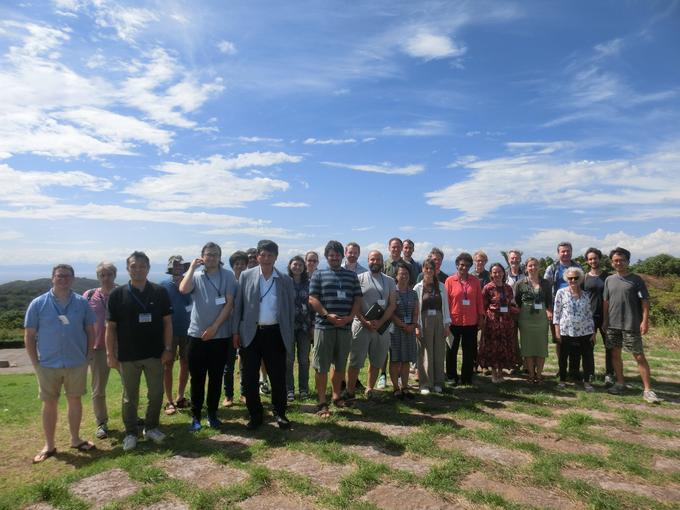
Overview
Description of the Meeting
The main objective of the proposed meeting is to identify and explore key emerging connections between two active algorithmic research areas: graph rigidity theory, with applications in diverse geometric modeling scenarios and algebraic statistics, with applications in data-driven inference and machine learning. To this end, the invited experts in each field will outline recent fruitful interactions as well as mathematical and computational tools for the fundamental problems in these two areas to establish a common language for participants with different expertise.
Graph Rigidity Theory and Algorithmic Characterizations
A fundamental topic in computational geometry is the efficient algorithmic characterization of basic properties of point configurations in Euclidean space that satisfy various algebraic geometric constraints. Example properties of such feasible sets of point configurations are emptiness, finiteness, singularity, etc. Rigidity theory establishes an underlying mathematical foundation by revealing and studying discrete structures, both geometric and purely combinatorial, inherent in geometric constraint systems. This is a classical topic in discrete geometry, whose history can be traced back to the work of Euler, Cauchy, and Maxwell on the rigidity of polyhedra and skeletal frames. In the last two decades, both geometric and combinatorial rigidity theory have become particularly active, drawing on diverse areas of mathematics and computer science and engaging with a growing range of modern applications, such as Computer-Aided-Design, molecular modeling, localization in sensor networks, and the distributed control of formations of autonomous agents. As a result, the area has gained significant international visibility as an active and interdisciplinary research area. In Spring 2021, the Fields Institute in Toronto, Canada, ran a 6-month thematic program on this topic (Geometric Constraint Systems, Framework Rigidity, and Distance Geometry) and an additional 2-month program will take place in summer 2023. Furthermore, a 6-month program is planned at ICERM, Brown, USA, in spring 2025 (Geometry of Materials, Packings and Rigid Frameworks).
Algebraic Statistics, Emerging Connections,Timeliness
Recently it has become increasingly clear that rigidity theory and the similarly flourishing field of algebraic statistics – which employs algebraic approaches to data-driven, statistical, and machine learning questions – share common goals in capturing hidden discrete structures inherent in algebraic relations. Techniques in one field have been shown to shed new light on a challenging problem in the other field. One representative example is the estimation of the probability distribution for a sample of observations from a problem domain. Maximum likelihood estimation (MLE) is a common framework used throughout the field of machine learning. MLE involves maximizing a likelihood function in order to find the probability distribution and parameters that best explain the observed data, and hence, provides a framework for predictive modeling in machine learning where finding model parameters can be formulated as an optimization problem. Recently, rigidity theory has been shown to provide useful tools in the realm of Bayesian statistics and Gaussian graphical models. For example, it is shown that the maximum likelihood threshold of a graph, which is the smallest number of data points that guarantees that MLE exists almost surely in the Gaussian graphical model, is closely connected to rigidity theory.
In view of this emerging interaction, it is timely to hold an international workshop in 2024 whose focus and objective are to identify and explore key connections between rigidity theory and algebraic statistics. To this end, the invited experts in each field will outline recent mathematical and computational tools for the fundamental problems in graph rigidity or algebraic statistical inference and modeling, to establish a common language for participants with different expertise.
Problems in the Overlap Area of Algebraic Matroids
A key mathematical concept for facilitating such an interaction would be the theory of algebraic matroids. Matroid theory offers a general framework for analyzing discrete structures and developing efficient algorithms in various fields, such as combinatorial optimization and machine learning. Several key questions in rigidity theory and algebraic statistics are interrelated through formulations in terms of matroids associated with algebraic varieties. Thus the workshop aims to provide an occasion for researchers to share the state-of-art techniques for analyzing algebraic matroids and discussing future research directions. Specific problems we would like to address during the workshop are the following.
- Combinatorial and efficient algorithmic characterization of independence/dependence in a variety of algebraic matroids: efficient algorithms will be sought for, e.g. checking consistency or redundancy of geometric constraint systems or algebraic relations that capture specific data properties.
- Geometric and efficient algorithmic characterization of the identifiability of algebraic systems in terms of independence/dependence in algebraic matroids: efficient algorithms will be sought for, e.g. checking completeness of algebraic constraint systems and the identifiability of data properties from partial measurements from experiments.
- Development of a common framework for identifying underlying algebraic matroids in applications: this contributes to an acceleration of applications and a systematization of the theory of algebraic matroids.