NO.206 Human-Centered Machine Translation
March 11 - 14, 2024 (Check-in: March 10, 2024 )
Organizers
- Marine Carpuat
- University of Maryland, College Park, USA
- Toru Ishida
- Hong Kong Baptist University, Hongkong SAR
- Niloufar Salehi
- University of California, Berkeley, USA
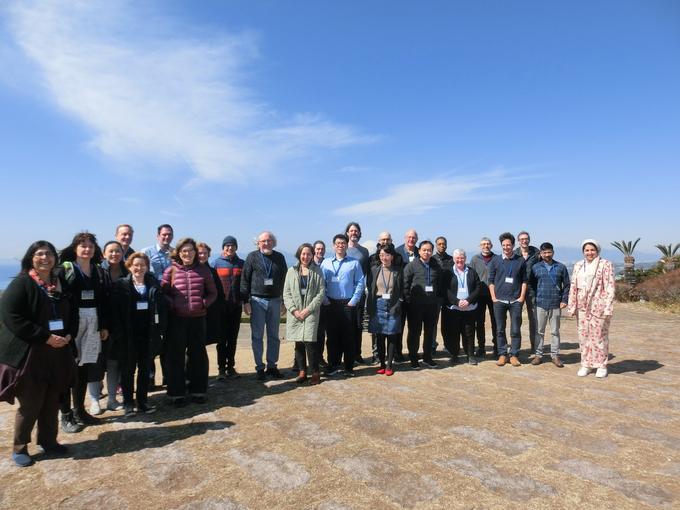
Overview
Project Description
Despite advances that have made Machine Translation (MT) seemingly universally available to anyone with internet access, progress has had a disparate impact across user populations, and MT remains unable to meet the wide range of needs for cross-lingual communication. State-of-the-art systems perform unequally across languages and domains, and they make errors that are opaque and counter-intuitive. This hinders access for users who have limited or no fluency in their non-native languages, and makes it difficult for lay users to make informed assessments of translation quality before deciding whether and how to rely on MT. For example, MT is not reliable enough to support migrant workers when seeking employment, navigating employer relations, or looking for healthcare information (Liebling et al., 2020). At the same time, high expectations about MT capabilities lead some users to use and trust MT outputs even when they should not, including in high-stakes settings (Asscher and Glikson, 2021; Vieira et al., 2021; Rossetti et al., 2020).
For MT to actually benefit users across all segments of society, we envision a human-centered approach to machine translation, which broadens the scope of what an MT system is expected to do to allow users to weigh the benefits of MT against the risks it may pose, and situates MT research in specific use cases, so that risks caused by mistranslations can be better characterized and inform MT research directly. Designing such human-centered systems requires an interdisciplinary approach, bringing together expertise in machine translation, natural language processing, human-computer interaction, translation studies and communication.
The time is ripe for fostering such an interdisciplinary collaboration. Recent advances have led machine translation researchers to rethink how to evaluate MT systems (Bawden et al., 2021; Fomicheva et al., 2020), present outputs to users (Zouhar et al., 2021), explore privacy considerations (Hisamoto et al., 2020), account for translation variability (Miyata and Fujita, 2021; Mayhew et al., 2020) and provide control mechanisms on outputs (Niu et al., 2017; Post and Vilar, 2018; Agrawal and Carpuat, 2019). However much of this research is disconnected from use cases, and would benefit from insights and methodology from HCI and translation studies to address stakeholder needs more directly. Meanwhile, a wealth of studies highlight the potential and limitations of technology to overcome language barriers from a human-centered perspective (Deng et al., 2022; Liebling et al., 2021; Pituxcoosuvarn et al., 2020; Bowker and Ciro, 2019; O’Brien et al., 2018; Gao et al., 2015, 2014; Yamashita et al., 2009; Yamashita and Ishida, 2006). However, these studies often rely on black-box MT systems and tools, which limits the scope of the research questions and the design solutions considered. We seek to learn from successful interdisciplinary collaborations (Green et al., 2015; Zhang et al., 2021, for instance) and broaden their scope and impact by fostering a larger community.
By bringing together for the first time researchers from all relevant fields, this workshop will lay the foundation for a research agenda that designs-in stakeholders needs when developing technology to break language barriers. The organizers bring complementary expertise in machine translation and multilingual natural language processing (Carpuat), technology-enabled intercultural collaboration (Ishida) and human-computer interaction (Salehi). We will invite diverse participants, spanning multiple disciplines, countries, seniority levels, and institutions to create an interdisciplinary human-centered machine translation research community.
We propose a standard 4-day Shonan meeting, starting with representative presentations of past research and a brainstorming session to identify open questions of interest to the group. While the specific questions that arise will depend on the interests of the participants, potential examples include: What dimensions of translation should be controllable by users and how? How should machine translation errors be explained to audiences of varying proficiency in the languages involved? How can we help users adequately calibrate their trust in machine translation? How to help users craft better inputs? How can we help users use imperfect outputs effectively? How to design machine translation for specific settings (e.g., healthcare, multilingual team work, education)? Our goal is to prompt conversations that will seed long-term interdisciplinary and international collaborations, leading to funding proposals, publications across disciplines, and initiatives to increase the public’s machine translation literacy throughout the world.
References
Sweta Agrawal and Marine Carpuat. 2019. Controlling Text Complexity in Neural Machine Translation. In Proceedings of EMNLP-IJCNLP.
Omri Asscher and Ella Glikson. 2021. Human evaluations of machine translation in an ethically charged situation. New Media & Society, page 14614448211018833.
Rachel Bawden, Eric Bilinski, Thomas Lavergne, and Sophie Rosset. 2021. DiaBLa: A corpus of bilingual spontaneous writ[1]ten dialogues for machine translation. Language Resources and Evaluation, 55(3):635–660.
Lynne Bowker and Jairo Buitrago Ciro. 2019. Machine Transla[1]tion and Global Research: Towards Improved Machine Trans[1]lation Literacy in the Scholarly Community. Emerald Publish[1]ing Limited.
Wesley Hanwen Deng, Nikita Mehandru, Samantha Robertson, and Niloufar Salehi. 2022. Beyond General Purpose Ma[1]chine Translation: The Need for Context-specific Empirical Research to Design for Appropriate User Trust.
Marina Fomicheva, Shuo Sun, Erick Fonseca, Frédéric Blain, Vishrav Chaudhary, Francisco Guzmán, Nina Lopatina, Lu[1]cia Specia, and André F. T. Martins. 2020. MLQE-PE: A Multilingual Quality Estimation and Post-Editing Dataset. arXiv:2010.04480 [cs].
Ge Gao, Bin Xu, Dan Cosley, and Susan R. Fussell. 2014. How beliefs about the presence of machine translation impact mul[1]tilingual collaborations. In Proceedings of CSCW.
Ge Gao, Naomi Yamashita, Ari M.J. Hautasaari, and Susan R. Fussell. 2015. Improving Multilingual Collaboration by Dis[1]playing How Non-native Speakers Use Automated Transcripts and Bilingual Dictionaries. In Proceedings of CHI.
Spence Green, Jeffrey Heer, and Christopher D. Manning. 2015. Natural Language Translation at the Intersection of AI and HCI: Old questions being answered with both AI and HCI. Queue, 13(6):30–42.
Sorami Hisamoto, Matt Post, and Kevin Duh. 2020. Membership Inference Attacks on Sequence-to-Sequence Models: Is My Data In Your Machine Translation System? Transactions of the Association for Computational Linguistics, 8:49–63.
Daniel J. Liebling, Katherine Heller, Margaret Mitchell, Mark Díaz, Michal Lahav, Niloufar Salehi, Samantha Robertson, Samy Bengio, Timnit Gebru, and Wesley Deng, editors. 2021. Three Directions for the Design of Human-Centered Machine Translation.
Daniel J. Liebling, Michal Lahav, Abigail Evans, Aaron Dons[1]bach, Jess Holbrook, Boris Smus, and Lindsey Boran. 2020. Unmet Needs and Opportunities for Mobile Translation AI. In Proceedings of CHI, CHI ’20.
Stephen Mayhew, Klinton Bicknell, Chris Brust, Bill McDowell, Will Monroe, and Burr Settles. 2020. Simultaneous Transla[1]tion and Paraphrase for Language Education. In Proceedings of the Workshop on Neural Generation and Translation.
Rei Miyata and Atsushi Fujita. 2021. Understanding Pre-Editing for Black-Box Neural Machine Translation. In EACL.
Xing Niu, Marianna Martindale, and Marine Carpuat. 2017. A Study of Style in Machine Translation: Controlling the For[1]mality of Machine Translation Output. In EMNLP.
Sharon O’Brien, Michel Simard, and Marie-Josée Goulet. 2018. Machine Translation and Self-post-editing for Academic Writ[1]ing Support: Quality Explorations. In Joss Moorkens, Sheila Castilho, Federico Gaspari, and Stephen Doherty, editors, Translation Quality Assessment: From Principles to Practice, Machine Translation: Technologies and Applications, pages 237–262. Springer International Publishing, Cham.
Mondheera Pituxcoosuvarn, Yohei Murakami, Donghui Lin, and Toru Ishida. 2020. Effect of Cultural Misunderstanding Warn[1]ing in MT-Mediated Communication. In Collaboration Tech[1]nologies and Social Computing, Lecture Notes in Computer Science.
Matt Post and David Vilar. 2018. Fast Lexically Constrained De[1]coding with Dynamic Beam Allocation for Neural Machine Translation. In Proceedings of ACL.
Alessandra Rossetti, Sharon O’Brien, and Patrick Cadwell. 2020. Comprehension and Trust in Crises: Investigating the Impact of Machine Translation and Post-Editing. In EAMT.
Lucas Nunes Vieira, Minako O’Hagan, and Carol O’Sullivan. 2021. Understanding the societal impacts of machine transla[1]tion: A critical review of the literature on medical and legal use cases. Information, Communication & Society, 24(11):1515– 1532.
Naomi Yamashita, Rieko Inaba, Hideaki Kuzuoka, and Toru Ishida. 2009. Difficulties in establishing common ground in multiparty groups using machine translation. In CHI. Naomi Yamashita and Toru Ishida. 2006. Effects of machine translation on collaborative work. In Proceedings of CSCW.
Yongle Zhang, Dennis Asamoah Owusu, Emily Gong, Shaan Chopra, Marine Carpuat, and Ge Gao. 2021. Leveraging Machine Translation to Support Distributed Teamwork Be[1]tween Language-Based Subgroups: The Effects of Automated Keyword Tagging. In Extended Abstracts of CHI.
Vilém Zouhar, Michal Novák, Matúš Žilinec, Ondˇrej Bojar, Ma[1]teo Obregón, Robin L. Hill, Frédéric Blain, Marina Fomicheva, Lucia Specia, and Lisa Yankovskaya. 2021. Backtranslation Feedback Improves User Confidence in MT, Not Quality. In Proceedings of NAACL