NO.200 Social Explainable AI: Designing Multimodal and Interactive Communication to Tailor Human–AI Collaborations
September 18 - 21, 2023 (Check-in: September 17, 2023 )
Organizers
- Kary Främling
- Umeå Universitet, Sweden
- Brian Y. Lim
- National University of Singapore, Singapore
- Katharina J. Rohlfing
- Paderborn University, Germany
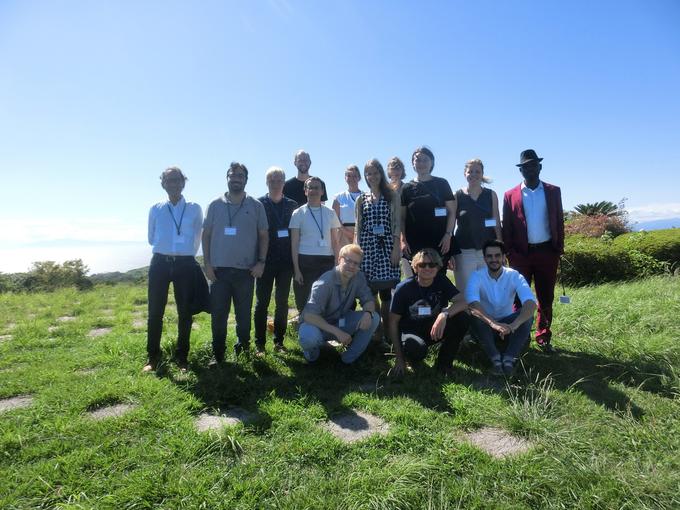
Overview
Motivation
In our digitized society, algorithmic approaches (such as machine learning or autonomous intelligent systems) are rapidly increasing in complexity, making it difficult for citizens to understand their assistance and to accept the decisions they suggest. In response to this societal challenge, research on explainable AI (or XAI) has intensified, pushing forward many ideas of how algorithms should be explainable or even be able to explain their own output. Consequently, recent work on XAI has broadened its perspective, tackling topics such as verbal explanations, hybrid approaches combining reasoning and learning for XAI, and relevance of explanations to the users.
Whereas the emerging XAI approaches are concerned with interpretability or explainability, more recently, state-of-the-art research reveals a lack of context-awareness [1], lack of interaction, and personalization as reasons for why an explainable system is little useful to the users [2]. It seems that an explanation does not necessarily lead to an understanding. Instead, the interaction of current XAI systems is severely limited because they can only deliver an explanation, without tailoring it to the receivers’ understanding, their informational needs, or the given context. Responding to this limitation, Miller [3] argues that explainable AI can benefit from social science research. In this line of research, interaction seems to be the key making an explanation provided by a system understandable and relevant. However, instead of being a generic exchange, Rohlfing and colleagues [4] propose that interaction should follow specific patterns in order to serve the purpose of an explanation—a social design of XAI.
Aim
In this meeting, we will gather scholars from different disciplines (economics, linguistics, philosophy, psychology, sociology) to account for the question of how explanation generation can be tailored. We propose that, rather than being ‘delivered’ by the explainer, explanations become tailored when they emerge at the interface between explainer and explainee, who are both active participants shaping explanations during a social interaction. This constructive process of participating in an interaction, however, is ultimodal. Thus, multimodal signals of communication need to be regarded when explanations are tailored and gain relevance for the users.
To foster a social design of Explainable AI, research needs to acknowledge three facts concerning social interaction in general and explanatory dialogues specifically:
- Interaction is multimodal (e.g., visual, verbal, auditory), so XAI needs to account for different modalities of communications that are used in the process of constructing an explanation [1].
- Interaction is incremental and builds on the contribution of the involved partners who adapt to each other. In this sense, an explainee can also contribute to a successful explanation, e.g., by asking questions or by providing feedback of understanding [2].
- Interaction is patterned in the sense that different contexts and goals will lead to the emergence of different social roles impacting the construction of explanations. In the case of an explanation, specific conversational patterns of, e.g., explicating the relations, are followed [4].
Objectives and expected outcomes
In addressing the first fact (Interaction is multimodal), we will identify important social signals that need to be considered in an interactive paradigm of human-machine interaction for the purpose of XAI. Adhering to the second fact (Interaction is incremental), we will critically discuss first approaches in which an XAI constructs an explanation with a human partner: What are their benefits and drawbacks? With respect to the third fact (Interaction is patterned), we will invite scholars from other disciplines to cast light into patterns being established within a particular context (e.g., healthcare, finance) that yield specific dialogical roles influencing explanations but also understanding.
With these objectives and planned activities, we aim to raise the awareness for a social design of XAI within the participating scholars, and we propose a road map for a social design of XAI that will be published to keep the community informed suggesting specific next steps. In addition, the group will establish a schedule for further activities (special issues, conferences, panels) in 2024 and 2025.
Significance and innovation
The proposed meeting will extend current research in computer science and offer new answers to the abovementioned societal challenge by contributing to the development of: (a) a multidisciplinary understanding of the mechanisms involved in the process of explaining, tailoring it to the process of understanding, (b) computational models and complex AI systems that focus efficiently on what kind of explanation a person requires in a current context, and by (c) interacting multimodally to achieve an interaction that unfolds over time and that makes a joint construction of an explanation possible.
Plan for Workshop Program
Day 1:
Invited Talks on XAI and social design session (organizers)
Day 2:
Working groups on aspects of the social design of XAI: Multimodality, Adaptivity, Patternedness
Day 3 (usually only half a day):
Writing a road map along the aspects of the social design for XAI
Day 4 (usually only half a day):
Working groups’ presentations and planning for continuation of the research (joint schedule of further activities)
[1] Anjomshoae, S., Najjar, A., Calvaresi, D., & Främling, K. (2019). Explainable agents and robots: Results from a systematic literature review. In 18th International Conference on Autonomous Agents and Multiagent Systems (AAMAS 2019), (pp. 1078–1088).
[2] Sokol, K., & Flach, P. (2020). One explanation does not fit all. KI-Künstliche Intelligenz, 34(2), 235–250.
[3] Miller, T. (2019). Explanation in artificial intelligence: Insights from the social sciences. Artificial Intelligence, 267, 1–38.
[4] Rohlfing, K. J., Cimiano, P., Scharlau, I., Matzner, T., Buhl, H. M., Buschmeier, H., ... & Wrede, B. (2020). Explanation as a social practice: Toward a conceptual framework for the social design of AI systems. IEEE Transactions on Cognitive and Developmental Systems, 13(3), 717–728.