NO.196 Everlasting Social Signal Processing - From Labs into the Real World
March 13 - 16, 2023 (Check-in: March 12, 2023 )
Organizers
- Sozo Inoue
- Kyushu Institute of Technology, Japan
- Wolfgang Minker
- Ulm University, Germany
- Björn Schuller
- Imperial College London, UK / University of Augsburg, Germany
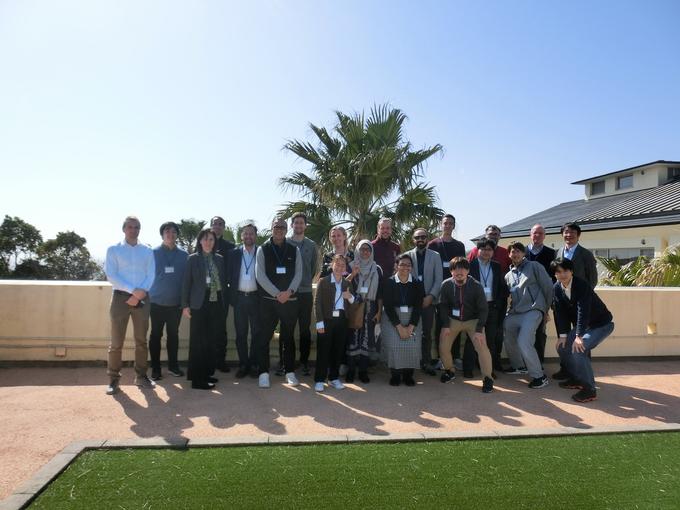
Overview
*Important: the venue is changed to Shonan OVA from the original site, Shonan Village Center.
Social signals play an indispensable role in our lives. To understand their importance, we can note the first emotions a child experiences soon after birth. Negative social signals expressed in shouting, crying, and hand waving, perform a protective or communication function, reflecting some kind of trouble for a baby: hunger, sickness, desire to sleep or play, etc. When growing up, the range of signals experienced by a person expands significantly, while at the same time improves his or her ability to correctly understand those signals from people around.
Social Signal Processing (SSP) is a new research domain that attempts to comprehend and to predict social interactions and to provide computers with similar skills in human-computer interaction situations. For computers, the task of Social Signal Processing remains difficult, especially when it comes to real-world conditions. Nevertheless, the rapid development of pervasive and ubiquitous computing technologies, machine learning algorithms in recent years suggests that machines will soon be able to reliably perform this task as well. Nowadays, a tremendous number of intelligent devices and objects, including smart speakers, watches, fitness trackers, microwaves, smartphones, scales, etc., surrounds us. They all perform calculations and collect information. Every single day more such devices emerge and form the basis of what we describe as “smart environments”. These should enable us to perform pervasive and ubiquitous computing. Thanks to the strong and recent development of artificial intelligence, the range of tasks that machines may be able to solve has expanded significantly. However, the problem of Social Signal Processing, which is important in human-computer interaction, for instance, in multimodal dialogue systems, remains challenging since the majority of the experiments are carried out under clean and neat laboratory conditions.
Moving on to Social Signal Processing from labs in real-world conditions, we are facing novel types of challenges at each of the different stages of data analysis including (long-term) data collection, evaluation, storing, and protection of the applied models. All tasks that have successfully been resolved in the laboratory may encounter significant burdens when transiting into the wild whilst applying an identical set up and the same approaches as before. Difficulties may already arise at the stage of setting up the experiment. When conducting experiments in the wild over a long period of time, it becomes necessary to take into account all external factors that may affect the results of such an experiment. This can be attributed to the stages of feature engineering and selection. Maintenance of the participants’ motivation poses another problem. For example, during negative emotional experiences, participants may not attach proper importance to the experiment and skip filling in questionnaires, which leads to poor quality of the collected data. Another weak point seems to be the equipment itself that is used to carry out the research. While using complex and professional devices in laboratories does not cause any significant problems, the possibility of using those under real conditions may be rather limited. The question arises about finding analogs to the original signal sources that will provide a comparable signal quality. The examples described above do not cover all the problems, but should reflect their complexity.
The aim of this Shonan meeting is therefore to discuss challenges that may arise when transiting Social Signal Processing from laboratory conditions into real environments and to propose suitable solutions as well as to establish a roadmap.
To move closer to the solutions of the challenges described above, significant efforts are required from the scientific community involving specialists in the field of computer science, medicine, and psychology. Sharing and combining expertise from various scientific fields may lead to synergies, which will allow us to create new ways to build solutions or increase the effectiveness of existing approaches. The Shonan Meeting will help to explore possible challenges and jointly develop a research agenda for main directions. Therefore, we will invite keynote speakers from the respective research fields, whose contributions will serve as a basis for breakout sessions. In these sessions, participants will work actively on specific research objectives in small groups. This will help foster an interdisciplinary understanding and cooperativity. The results of the breakout sessions will then be discussed with the whole plenum.
We have decided to structure the workshop into four different areas. These include typical research challenges that need to be addressed when transiting Social Signal Processing from laboratory conditions into the wild. Secondly, typical issues of development, testing and evaluation will be discussed. Suitable use cases and industrial applications will be covered as well. Finally, ethics and societal impact need to be considered.
Research challenges:
- Experimental set-up accounting for real-world, real-time, large-scale conditions.
- Data robustness: suppressing noise and handling missing data.
- Data quality: Reliable annotation and motivation of participants.
- Feature engineering and modality selection for Social Signal Processing (SSP) taking into account the environment.
- Reliable physiological signal processing.
- Energy, memory, and computing efficient SSP. Model pruning/shrinking for mobile use.
- From stationary to mobile systems performing SSP. Cloud vs. Edge computing.
- Efficient embedding of SSP.
- Protected data operating, such as federated learning.
- Green social signal processing protecting our environment.
- Virtual and Augmented Reality for SSP to simulate real-world conditions.
- Human-Computer Interaction making use of SSP.
- Data efficient learning and adaptation such as by reinforcement learning.
- Detection of social signal hiding.
- Real-time hiding and conversion of social signals.
- Adversarial attacks and methods to resist.
- Trust and usability.
Development, testing and evaluation:
- Experimental design, user studies and evaluation of socially empowered systems.
- Engineering approaches to SSP: life-cycle, requirement elicitation, robustness to change, standardization and simulation.
- Development models, tools and strategies: middleware, tools, languages.
- Meaningful and explainable system evaluation
- Description, development and sharing of resources: corpora compilation, annotation tools and approaches, crowdsourcing approaches.
- Pervasive sensing devices and frameworks, the Internet of Things and its relation to SSP.
- Competitive research challenges planning and organisation
Use cases and industrial applications:
- Computer-mediated human-to-human interaction
- Security applications
- Health applications
- Tutoring and E-learning applications
- Entertainment and Gaming
- Social Human-Robot Interaction
- Success stories, functional systems and industrial challenges.
Ethics and societal impact:
- Social responsibility.
- Data protection and privacy by design and default.
- Legal issues.
The outcome of the workshop will be published in the form of free open-access (http://ceur-ws.org/). This is expected to encourage joint publications at top conferences and journals in computer science, jointly authored by psychologists, ethicists, and AI researchers.