NO.179 Computational metabolomics and machine learning
May 8 - 11, 2023 (Check-in: May 7, 2023 )
Organizers
- Sebastian Böcker
- Friedrich Schiller University Jena, Germany
- Hiroshi Mamitsuka
- Kyoto University, Japan
- Juho Rousu
- Aalto University, Finland
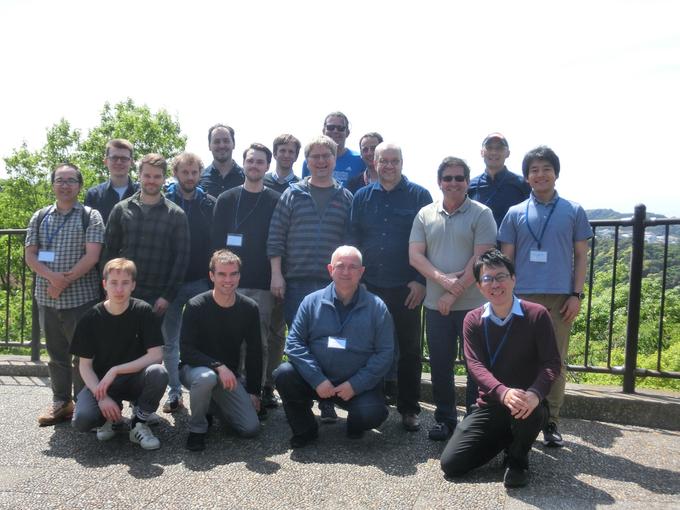
Overview
Description of the Meeting
*Important: the venue is changed from Shonan Village Center to Shonan OVA (update on January 30, 2023).
In recent years, machine learning has emerged and important tool in the research of small molecules, aiding their identification from measurement data, deciphering their interactions with proteins and other small molecules, and helping to elucidate the inner workings of cellular machinery. The research on the small molecule complement of the genome, metabolomics, has been referred to as the apogee of the omics-sciences, as it is closest to the biological phenotype. Metabolites are not only responsible for tasks such as growth, development, and reproduction, but also directly relevant to structure, signaling, and chemical interactions with other organisms. Most pharmaceuticals are small molecules that bind to their targets, thus altering their behavior.
In small molecule identification, mass spectrometry is the predominant analytical technique for detecting and identifying metabolites and other small molecules in high-throughput experiments. Huge technological advances in mass spectrometers and experimental workflows during the last decades enable novel investigations of biological systems on the metabolite level. But these advances also resulted in a tremendous increase of both amount and complexity of the experimental data, and “making sense” of the data is among the most pressing issues in high-throughput settings. Machine learning methods for small molecule identification have made great progress during the last decade, however, the identification problem is still far from being “solved”.
In drug discovery, several high-throughput anticancer drug screening efforts have been conducted, providing drug interaction and response measurements that allow for the identification of compounds that show increased efficacy in specific human cancer types or individual cell lines, therefore guiding both the precision medicine efforts as well as drug repurposing applications. Machine learning methods have shown their potential in these tasks, in e.g. several recent DREAM challenges organized around the theme. Similarly, in functional genomics, the prediction of biosynthetic gene clusters through machine learning is an active topic, concerned with the elucidation of the metabolites associated with a biosynthetic pathway of an organism.
During the last decade, metabolomics has seen co-operations developing between experimental and computational scientists. In turns out that the interpretation of the data is highly challenging; and as soon as one goes beyond the presence or absence of peaks in MS1 experiments, methods which have been developed in genomics or proteomics cannot be applied to metabolomics data. In particular, the application of machine learning techniques is impeded by several issues: For example, metabolites are graphs and representing them for machine/deep learning algorithms requires special care (e.g. string-based techniques such as LSTM neural networks are not directly applicable). Also, available training data for small molecules is usually very far from being a representative, even less so a uniform subsample of the complete space of molecules. Thus generalization outside the current data is challenging and machine learning methods are prone to overfit.
The key goal of this seminar is to foster the exchange of ideas between bioinformatics and machine learning for small molecules. State-of-the-art methods from computer science, statistics, analytical and biological experiments will be presented, along with problems arising from these techniques. Brainstorming sessions and break-out groups will discuss individual topics in greater detail, to initiate new collaborations between participants who have not yet worked together. This exchange of expertise is needed to form a scientific community to advance computational metabolomics. Ultimately, novel algorithms and methods are to be developed that will advance metabolomics as a whole. We will invite to the workshop a diverse group of scientists working in the fields of machine learning, bioinformatics, metabolomics and drug discovery, including both leading names in their fields as well as young rising talent.
A selection of topics to initiate discussions at the seminar include:
- Representation of small molecules: Can we learn better representations from data than the existing fingerprint definitions?
- Identifying “unknown unknowns”: How can we predict new molecular structures that are not in any training data set. Can zero-shot learning methods help here? Can we develop algorithms and databases for hypothetical metabolites?
- Linking molecular dynamics simulations to existing frameworks: Can we use molecular simulation to augment machine learning approaches?
- Integration of orthogonal data (Retention time, CCS): When and if it helps small molecule identification?
- From metabolome to systems: How can we best link predicted metabolites to the underlying biological pathways and networks? Can we develop methods to help to "look" for metabolites rather than performing non-target identification?
- Identification statistics: How can we compute statistics to improve identification quality of metabolites, such as False Discovery Rates? Can we find methods to compute decoy databases?
- Data exchange and public reference data: How can metabolomics researchers be encouraged to provide additional training data that covers a sufficient breadth of the expected molecular space? How can we obtain data that reasonably unbiased for machine learning?
- Searching in molecular structure databases: How can the promising approaches MetFrag, MAGMa, FingerID, CFM-ID and others be improved? As the underlying problems are similar to Graph Motif and also NP-hard, how can we avoid an explosion of running times?
- Experimental frontiers: Incorporation of experimental strategies such as data-independent acquisition (DIA), ultrahigh resolution, imaging mass spectrometry, 2-dimensional chromatography etc. in metabolomics.