NO.150 Learning to Communicate: Challenges in Language Learning by AI, Robots and Humans
February 18 - 22, 2019 (Check-in: February 17, 2019 )
Organizers
- Michael Spranger
- Sony Computer Science Laboratories Inc., Japan
- Tadahiro Taniguchi
- Ritsumeikan University, Japan
- Angelo Cangelosi
- AIST AIRC, Japan & The University of Manchester, UK
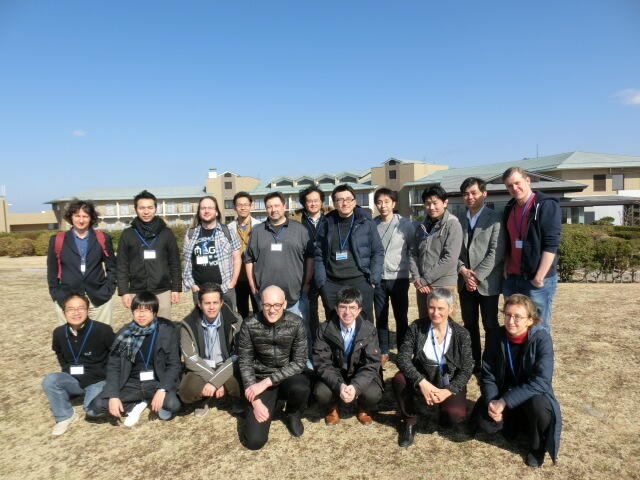
Overview
Communication emerges in humans through interaction with other agents in a physical, social and cultural environment. These interactions require a high level of sensory-motor intelligence (visual perception, body movement, navigation, object manipulation, auditory perception and articulatory control) but at the same time further enhance the capabilities and skills of the individual. Importantly, communication is based on representations and skills that start to develop earlier and that are shaped already by first social interactions. Continuously, representations become further enriched in ongoing interactions and across different contexts. All this appears to happen almost effortlessly in humans. How does this work? What are algorithms and representations that allow machines to become fluent learners, participants and shapers of communication systems similar in complexity to human language. Even though there are various efforts in developmental robotics, natural language processing, machine learning and artificial intelligence (AI) to build communication machines, the interaction, communication and language remain unsolved problems. We are still lacking theories and implementations that show how cooperation, interaction and communication can develop in long-term experiments with populations of robotic agents and/or mixed human-robot environments.
Recent advances in natural language processing and deep learning in particular in visual QA (Krishna et al, 2017), semantic parsing (e.g. Lian el 2017), learning from explanations (Srivastava et al, 2017) offer important opportunities for building embodied language learning systems. Similarly, progress in deep reinforcement learning (DRL), especially applications of DRL to the emergence of language (e.g. Foerster et al 2016) offer new ways of understanding language learning in artificial systems. Robotics researchers are also tackling with and making progress in problems related to emergence of symbol systems (e.g. Taniguchi et al 2016).
The goal of the meeting is to discuss these various state-of-art methods and see how they can be used to build embodied language learning systems. The meeting is not restricted to a particular set of computational approaches but takes an inclusive approach examining various representations (neural networks, probabilistic and symbolic models) and modeling schemes (supervised learning, reinforcement learning, language games etc). Addressing language learning requires much more than just AI and Machine Learning combining and integrating knowledge from diverse disciplines. The goal of this meeting is to bring together researchers from diverse areas in order to discuss current findings from experimental and computational studies and to inspire new experiments, algorithms and models. In particular, the meeting aims to develop new research agendas and roadmaps for the following topics.
- The ability to cooperate as well as to communicate is assumed to rely on rich embodied representations (visual, auditory and action). One of the key objectives of the meeting will be to understand possible joint representations (e.g., sensorimotor schemas and constructions). In particular the meeting will focus on the overlap in these representations and on the question how such representations can serve different tasks as in motor control and linguistic communication or when recruited in communication.
- Language is grounded in social environments and in particular in cooperative tasks and joint action. There are often multiple strategies (gestural, interactional etc) relying on mutual beliefs, emerging interaction patterns etc that together enable agents to solve cooperative tasks. How can we develop models that allow for the common understanding of language to emerge while agents engage in cooperative tasks? What is the relationship and impact of comnucative interactions with respect to mutual beliefs, theory of mind etc.
- The meeting will examine cognitive and computational architectures for learning to interact, communicate and use natural language including the learning of acquisition strategies themselves (meta-learning). A special emphasis will be on architectures that enable the interplay of different strategies on all levels of development e.g. the acquisition and evolution of interaction patterns, shaping and change of word meanings, schematisation of syntax and semantics etc.
- Language is learned in communicative environments that are themselves constructed by members of the population to solve cooperative tasks. How does cooperation, interaction and communication co-evolve? The meeting will also examine recent advances in agent-agent models of the development of interaction, communication and language (for example in reinforcement learning and cultural evolution settings.)
The problem of language requires an interdisciplinary approach. This meeting aims to bring together researchers with broad expertise in various fields―developmental robotics, robotics, machine learning, computer vision, natural language processing, neuroscience, linguistics and psychology―to discuss the state-of-the-art and develop new perspectives and future directions. The scope of this meeting is language learning.
- Human language learning
- Computational and robotic approaches to language acquisition
- Long-term adaptation and learning of language in robots and humans
- Visually (and sensorimotor) grounded dialogue (visual QA)
- Language learning and motivational systems (intrinsic motivation, curriculum learning)
- Development of communication and cultural language evolution in robot populations using language games (Spranger, 2016) and deep reinforcement learning (Forester2016)
[References]
Liang, Chen, et al. “Neural symbolic machines: Learning semantic parsers on freebase with weak supervision.” Proceeding of the 55th Annual Meeting of the Association for Computational Linguistics (Volume 1: Long Papers), Association for Computational Linguistics, Vancouver, Canada (2017), pp.22-33.
Foerster, Jakob, et al. “Learning to communicate with deep multi-agent reinforcement learning.” Advances in Neural Information Processing Silver, David, et al. “Mastering the game of go without human knowledge.” Nature 550.7676 (2017): 354.
Krishna, Ranjay, et al. “Visual genome: Connecting language and vision using crowdsourced dense image annotations.” International Journal of Computer Vision 123.1 (2017): 32-73.
Srivastava, Shashank, Igor Labutov, and Tom Mitchell. “Joint concept learning and semantic parsing from natural language explanations.” Proceeding of the 2017 Conference on Empirical Methods in Natural Language Processing. 2017. Systems. 2016.
Spranger, Michael. The Evolution of Grounded Spatial Language. Language Science Press. 2016,
Tahiro Taniguchi, Takayuki Nagai, Tomoaki Nakamura, Naoto Iwahashi, Tetsuya Ogata, and Hideki Asoh, Symbol Emergence in Robotics: A Survey, Advanced Robotics, No.30 (11-12) pp. 706-728.