NO.137 From natural to artificial embodied intelligence: is Deep Learning the solution?
November 11 - 15, 2019 (Check-in: November 10, 2019 )
Organizers
- Lorenzo Jamone
- Queen Mary University London, United Kingdom
- Tetsuya Ogata
- Waseda University, Japan
- Beata Joanna
- University College London, United Kingdom
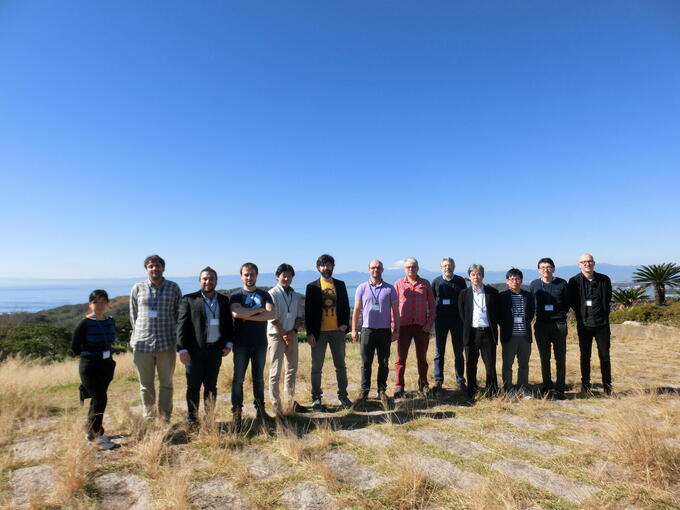
Overview
Description of the Meeting
Humans possess remarkable cognitive skills, acquired progressively over time through development and learning, that allow them to physically interact with the environment and to engage in social interactions.
Researchers have been attempting to model such cognitive capabilities in artificial systems for a long time now, first in computers [Newell1961,Russell1995] and more recently in robots as well [Lungarella2003, Asada2009]. Interestingly, the recent theories of embodied cognition [Anderson2003] and grounded cognition [Barsalou2008] have highlighted how the presence of a body, endowed with action and perception capabilities, is crucial for intelligence to emerge. In this light, robots have become even more interesting tools to understand human intelligence; at the same time, the positive impact that truly intelligent robots can have in our society is easy to foreseen and clearly impressive. However, although robots are becoming increasingly sophisticated, in terms of appearance, technology and motor skills, their interaction abilities are still very limited; this is true both for the interaction with the real unstructured environment (e.g. unknown objects and spaces) and even more dramatically for the interaction with people.
Notably, the world of Artificial Intelligence has been recently transformed by the increasing success of deep learning algorithms in many fields of applied computation: e.g., speech processing, image processing, data mining, finance, genomics [LeCun2015]. Powered by smart reinforcement learning strategies, deep neural networks are successfully playing video games exceeding human abilities [Mnih2013], and they have even beaten top professional human players at Go [Silver2016]. With the booming widespread interest in robotic technologies, it was natural to expect that researchers would have attempted to apply deep learning strategies to robot learning. So far, this has brought promising results, yet still in very limited settings, in particular for the learning of visuomotor mappings that would allow eye-hand coordination [Levine2016], grasping [Pinto2016], manipulation [Yang2017].
However, the breakthrough that most involved researchers are expecting is still to come.
One relevant aspect to consider is that the robotic interaction with the world (including both objects and people) is doomed by uncertainty (in action execution, action effects, perception, external events, etc…). Are deep learning paradigms able to cope with such unavoidable uncertainty?
Probabilistic approaches have been shown powerful results in complex robotic settings such as combined task and motion planning [Kaelbling2013] and problem solving from human instructions [Antunes2016], suggesting that recently proposed algorithms such as Deep Belief Networks [Lee2009] and Deep Variational Autoencoders [Kingma2013] could be promising solutions.
From a more general standpoint, it is not yet clear whether deep learning approaches could be the solution to some of the long-standing problems of artificial intelligence, as for example symbol emergence [Taniguchi2015], grounding [Harnad1990, Coradeschi2013] and anchoring [Coradeschi2003], and whether they could be a powerful addition to more general cognitive architectures [Vernon2016], that attempt to obtain artificial intelligence by combining insights from psychology, cognitive sicence and neuroscience with advanced computational tools.
Is deep learning going to revolutionize robotic intelligence in particular, and the robotics field in general? Are deep learning algorithms alone the solution to artificial embodied intelligence? Or is deep learning just a well performing computational tool to obtain good solutions for small sub- problems? How to combine such data-driven approaches with explicit modeling inspired by cognitive science and computational psychology theories? What is the role to be played by Cognitive Architectures? What is the role to be played by rule-based and symbol-based AI reasoning approaches?
This Shonan Meeting will attempt to answer these question (and to generate even more question possibly!). We will achieve that by gathering about 30-40 international senior researchers from different disciplines: cognitive science, experimental and developmental psychology, neuroscience, robotics, artificial intelligence, machine learning, human-robot and human-machine interaction. Following the successful experience of recent Shonan Meetings on related topics (in which some of the organisers and invited speakers of this proposal were participating), we will not have a detailed program for the five days, but we will actively drive the discussion from a very general initial theme (artificial embodied intelligence) to converge to a smaller and more focused topic (to be defined thorugh the discussion), the will be the subject of a joint publication to be prepared by all participants after the meeting (e.g. “Combining rule-based symbolic reasoning with deep sensorimotor learning for efficient human-robot collaboration: recent advances and promising directions”).
We believe that the interdisciplinary discussion among this group of established researchers with such diverse background can be a unique opportunity to identify the opportunities provided by the current technologies towards the goals of artificial intelligence, to stimulate new promising directions for future research, and to foster novel international collaborations with strong potential for innovation.
References:
[Newell1961] A. Newell and H. A. Simon, Computer simulation of human thinking, Rand Corporation, 1961.
[Russell1995] S. Russell and P. Norvig, Artificial Intelligence: A Modern Approach, Prentice Hall, Englewood Cliffs, NJ, 1995.
[Lungarella2003] M. Lungarella, G. Metta, R. Pfeifer and G. Sandini, Developmental robotics: a survey”. Connection Science 15(4), 151-190. 2003.
[Asada2009] M. Asada et al., “Cognitive Developmental Robotics: A Survey,” IEEE Transactions on Autonomous Mental Development 1(1), pp. 12-34, 2009.
[Anderson2003] M. L. Anderson, Embodied Cognition: A field guide, Artificial Intelligence 149(1): 91-130, 2003.
[Barsalou2008] L. W. Barsalou, Grounded cognition. Annu. Rev. Psychol., 2008. [LeCun2015] Y. LeCun, Y. Bengio and G. Hinton, Deep learning. Nature 521, 2015.
[Mnih2013] V. Mnih et al., Playing Atari with Deep Reinforcement Learning. ArXiv:1312.5602, 2013. [Silver2016] D. Silver et al., Mastering the game of Go with deep neural networks and tree search, Nature 529, 2016.
[Levine2016] S. Levine, C. Finn, T. Darrell and P. Abbeel, End-to-end training of deep visuomotor policies. Journal of Machine Learning Research, 17(39):1-40, 2016.
[Yang2017] P. C. Yang, K. Sasaki, K. Suzuki, K. Kase, S. Sugano, T. Ogata, Repeatable Folding Task by Humanoid Robot Worker Using Deep Learning. IEEE Robotics and Automation Letters 2 (2):397-403, 2017.
[Pinto2016] L. Pinto and A. Gupta, Supersizing self-supervision: Learning to grasp from 50K tries and 700 robot hours. ICRA 2016.
[Taniguchi2015] T. Taniguchi, T. Nagai, T. Nakamura, N. Iwahashi, T. Ogata, H. Asoh, Symbol Emergence in Robotics: A Survey, Advanced Robotics 30(11-12), 2016.
[Harnad1990] S. Harnad, The symbol grounding problem. Physica D: Nonlinear Phenomena 42(1), 1990.
[Coradeschi2013] S. Coradeschi, A. Loutfi and B. Wrede, A Short Review of Symbol Grounding in Robotic and Intelligent Systems. Künstl Intell 27: 129, 2013.
[Coradeschi2003] S. Coradeschi and A. Saffiotti, An introduction to the anchoring problem. Robotics and Autonomous Systems 43: 85-96, 2003.
[Vernon2016] D. Vernon, C. von Hofsten, L. Fadiga, Desiderata for developmental cognitive architectures, Biologically Inspired Cognitive Architectures 18:116-127, 2016.
[Kaelbling2013] L. P. Kaelbling, T. Lozano-Pérez, Integrated task and motion planning in belief space. The International Journal of Robotics Research 32:(9-10), 2013.
[Antunes2016] A. Antunes, L. Jamone, G. Saponaro, A. Bernardino and R. Ventura, From Human Instructions to Robot Actions: Formulation of Goals, Affordances and Probabilistic Planning. IEEE ICRA 2016.
[Lee2009] H. Lee, R. Grosse, R. Ranganath, A. Y. Ng, Convolutional Deep Belief Networks for Scalable Unsupervised Learning of Hierarchical Representations, ICML 2009.
[Kingma2013] D. P. Kingma and M. Welling, Auto-Encoding Variational Bayes. ArXiv:1312.6114, 2013.