NO.120 Visual Analytics: Towards Effective Human-Machine Intelligence
January 29 - February 1, 2018 (Check-in: January 28, 2018 )
Organizers
- Yingcai Wu
- Zhejiang University, China
- Nan Cao
- TongJi University, China
- Steffen Koch
- University of Stuttgart, Germany
- David Gotz
- UNC-Chapel Hill, USA
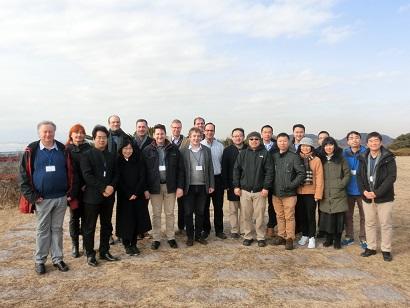
Overview
Data mining and visualization have attracted considerable attention in recent years for exploring and understanding big data. Machine learning focuses on developing automatic algorithms to discover patterns in large data sets. Although huge successes have been achieved, existing approaches usually assume that a ground truth is readily available. In practice, this is often not the case. In some cases, manual data annotation is required, which is tedious, onerous at scale, and highly dependent on the judgment of the human annotators. A ground truth understanding of a dataset may simply not exist, such that it can be difficult if not impossible to mathematically model the unknown types of patterns we hope to find. Even when patterns can be modeled, the intuitive explanation and validation of the models can pose a major challenge. Moreover, the underspecified complex tasks with a very high dimensional space of input variables and parameters cannot be simply handled without the inclusion of human expertise and knowledge. Compared with data mining, visualization aims to produce intuitive visual representations of data. It allows people to quickly see and interact with the patterns in the data by making effective use of their high-bandwidth visual system. As an old saying goes “a picture worth a thousand words”, a good visualization will significantly improve the abilities of people to understand and interpret the data and analysis results. However, the enormous amount of complex data leads to the difficulty of creating concise, discernable, and intuitive visual representations. Visual summaries of big data can still easily overwhelm users.
To make best use of the advantages and bypass the disadvantages of data mining and visualization, visual analytics has recently been introduced to facilitate analytical reasoning by interactive visual interfaces. It presents data and analysis results in context, and thus, it can provide rich evidence that supports or contradicts the analysis results, and consequently, help with data interpretation and result validation. Analysts can annotate on (e.g., place labels on) or adjust results via interactive visualizations to supervise the underlying analysis procedure, for example, and thus, gradually produce increasingly precise analysis and correct results. Visual analytics have been used in many applications to tackle various important problems, such as tackling urban issues like traffic jam and air
pollution, making better diagnostic and treatment decisions, preventing threats and fraud in business, optimizing rescue efforts, forecasting severe weather conditions, and achieving situational awareness during crisis.
We believe that visual analytics can enable human-centric computational intelligence by effectively integrating human knowledge and expertise into powerful computational algorithms through a high-bandwidth visual processing channel and user interactions. Despite recent impressive advances, designing developing effective visual analytics for big data still poses significant challenges for researchers and practitioners. There are still many research opportunities and open questions that we should address for creating visual analytics to enable effective human-machine intelligence.
- Uncertainty information: Either machines or humans can generate uncertainty. The integration of human knowledge and machine’s computational power through visual interfaces could further couple different kinds of uncertainty, which leads to the difficulty of coping with uncertainty. Enabling human-machine intelligence demands insights into the coupled uncertainty and better mechanisms to track and analyze the uncertainty.
- Collaborative analytics: The computational power of machines can be more easily scaled up with the advance of parallel computing technologies. However, scaling up human power by adding more people is still severely limited. It would be promising to explore how to scale up human power towards better collaborative human-machine intelligence.
- Immersive analytics: Interactive user interfaces are one of the keys to seamless integrate human knowledge and machine power. The recent advance of AR/VR techniques shed new light on how to enable highly effective and efficient human-machine intelligence. However, there remain many theoretical and technical challenges. New interaction models as well as design rules should be derived to better utilize those techniques for immersive analytics.
- Application adaption: Setting up mining and learning techniques requires considerable effort and expertise of expert users. Visual analytics could facilitate both the setup/creation of such approaches as well as their adaptation to similar scenarios and use cases.
- Situation-aware analytics: The analysis of dynamic data sources and streams such as telemetry data from vehicles, machinery, sensors, social media, computer network logs, etc. can require dynamic reaction by human users. It remains difficult to design intelligent and interactive visual analytics solutions to maintain situation awareness and make informed decisions in a timely manner.
- Algorithm transparency: Many learning and mining algorithms work as black boxes. It is often tedious and difficult for non-expert users to diagnose and debug the algorithms. Visual analytics can play important roles in making the algorithms more transparent by visually analyze input and result in a way that lets users infer some of the hidden workings. Nevertheless, the increasing
complexity of modern learning and mining algorithms (such as deep learning) makes such effort increasingly challenging. - Multi-source information: Big data can often be collected from multiple sources. For instance, urban data can have road networks, air quality data from air quality monitoring stations, weather data from weather stations, image/videos from surveillance cameras, geotagged microblog messages from social media, etc. The data from different sources are usually heterogeneous with rather diverse characteristics. The information can complement each other to uncover a full panorama of a city. However, visual analysis of the multi-source information demands new fundamental theories and techniques.
Aims and Objectives:
- We will identify research opportunities in visual analytics for enabling human-machine intelligence, with a focus on the Asia-Pacific context.
- We will bring together researchers that work in visualization and its interconnections to the research areas of machine learning and data mining to discuss and elaborate how interactive visualization and data mining needs to be integrated further.
- We will foster greater exchange between researchers and practitioners, and to draw more researchers in the Asia-Pacific region to enter this rapidly growing area of research.
Significance and Innovation:
It is one of the most significant problems to support human and machine to work together efficiently and effectively to solve many challenging problems which cannot simply be solved by machines as we discuss above. Innovative techniques for visual analytics for seamless integration of human and machines hold the most promise for the future. Visual analytics will be the disruptive and enabling techniques for researchers and end users in many application domains and other disciplines.
Expected Outcomes:
- Innovative theories, models, techniques, and solutions for visual analytics that enable human-machine intelligence. The innovation will inspire domain experts and end users in various application.
- Publications coauthored by visualization and/or machine learning researchers at the top conferences and journals in Visualization.