NO.116 Anonymization methods and inference attacks: theory and practice
March 5 - 8, 2018 (Check-in: March 4, 2018 )
Organizers
- Hiroaki Kikuchi
- Meiji University, Japan
- Josep Domingo-Ferrer
- Universitat Rovira i Virgili, Spain
- Sébastien Gambs
- Université du Québec à Montréal (UQAM), Canada
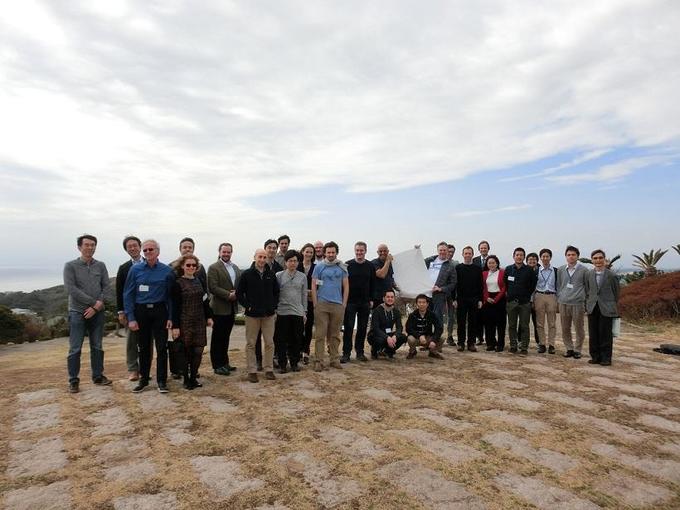
Overview
Overview
The democratization of mobile systems and the development of information technologies have been accompanied by a massive increase of the amount and the diversity of data collected about individuals. For instance, some actors have access to personal data such as social relationships, email content, income information, medical records, credit card and loyalty card usage, pictures taken through public and private cameras, personal files, navigation behaviour, or data issued from quantified self, just to name a few. On the one hand, the analysis of these large scale datasets, often refer to as Big Data, offer the possibility to realize inferences with an unprecedented level of accuracy and details. On the other hand, the massive collection of information raises many privacy issues since most of these large scale datasets contain personal information, which is thus sensitive by nature. As a result, only very few of them are actually released and available. This limits both our ability to analyze such data to derive information that could benefit to the general public and slows down the innovative services that could emerge from such data. It is therefore important to study anonymization mechanisms that can be used to remove the sensitive information or add uncertainty to a dataset before it is released or before further services are developed on it.
Designing an anonymization method that provides strong privacy guarantees while maintaining a high level of utility is known to be difficult task. In particular, pseudonymization is clearly not at alternative as illustrated by infamous examples of privacy failures such as the AOL release or the Netflix challenge. In addition, there is no free-lunch in anonymization and each type of data comes with its own challenges that have to be dealt with. For instance, to address appropriately the particularities of a genomic dataset, mobility traces or a social graph require the development of an anonymization method tailored to the specificities of the data considered. Nonetheless, defining realistic and formally grounded measures of privacy, which are adapted and appropriate for specific contexts, is a challenging task but also a prerequisite both for evaluating the risks and for assessing potential solutions.
One of the main difficulties is to be able to design and formalize realistic adversary models, by taking into account the background knowledge of the adversary and his inference capabilities. In particular, many privacy models currently exist in the literature such as k-anonymity, and its extensions such as l-diversity and t-closeness, or more recently differential privacy, pan-privacy and empirical privacy. However, these models are not necessarily comparable and what might appear to be the optimal anonymization method in one model is not necessarily the best one for a different model. To be able to assess the privacy risks of publishing a particular anonymized data, it is necessary to practically evaluate the accuracy inference attacks that can be performed by the adversary based on the released data but also on the possible background knowledge that he might have gathered. In addition, of the risk of re-identifying an individual, inference attacks can also target specific attributes. For instance, considering the example of location data, an inference attack can use the mobility data of a user, possibly with some auxiliary information, to deduce other personal data (home and place of work, main interests, social network, etc.), including sensitive data (in the legal sense) such as religion, health condition or business confidential data coming from the user’s employer.
The main objective of the proposed Shonan meeting is precisely to investigate the strengths and limits of existing anonymization methods, both from theoretical and practical perspective. More precisely, by confronting the points of views of privacy experts coming from diverse background such as databases, cryptography, theoretical computer science, machine learning, quantitative information, graph theory and social sciences, we aim at gaining an in-depth understanding on how to quantify the privacy level provided by a particular anonymization method as well as the achievable trade-off between privacy and utility of the resulting data. The outcomes of the meeting will greatly benefit to the privacy community and one of our objectives is to use them to design an international anonymization competition.