NO.085 Dynamic Networks Visual Analytics: Approaches to facilitate visual analysis of complex and dynamic network data.
August 1 - 4, 2016 (Check-in: July 31, 2016 )
Organizers
- Takayuki Itoh
- Ochanomizu University , Japan
- Karsten Klein
- University of Konstanz, Germany
- Beppe Liotta
- University of Perugia, Italy
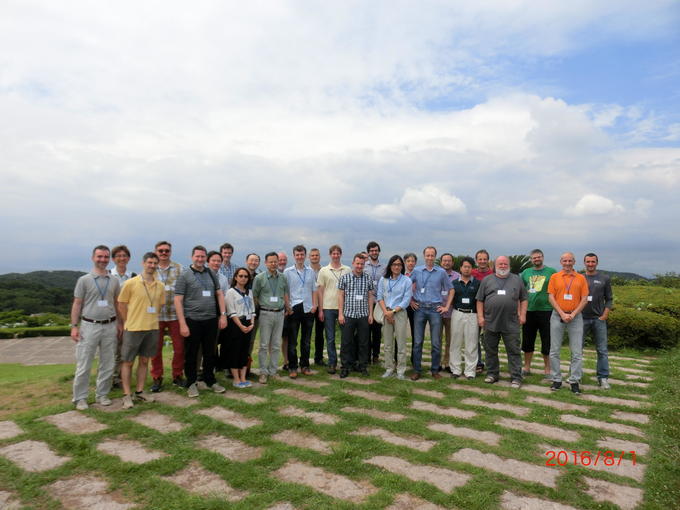
Overview
Description of the meeting
1. Introduction
There is an abundance of complex dynamic data across many domains. Often such data has a natural interpretation as a network, e.g. for applications in biology, the social sciences, telecommunication, finance, energy, and information security. Analysis of these dynamic networks is key to the understanding of complex processes both in fundamental research and for decision making. For example, neuroscientists try to unravel the inner workings of the human brain using activity correlation networks, regulators and finance companies investigate trading patterns at the stock market and their relation to information spread in social media, and energy providers monitor and analyze power generation, distribution, and consumption via sensor networks on electrical grids. Use-cases include real-time monitoring of events and processes as well as a posteriori analysis of recorded data to gain insight. This insight then can be used to explain the principles of an underlying system, to detect important artifacts, e.g. cases of emergency, or to predict future changes. Many of these cases require a human in the loop to make decisions, e.g. in emergency situations, or to allow experts to guide exploration and insight generation for complex processes. Lack of sufficient a priori knowledge, in combination with the complexity of the dynamics in multi-variate data hamper the use of purely rule-based systems for analysis and decision making. Thus, a visual analytics type approach is best suited for such cases. Efficient tools and methods for the visual analysis of network changes will facilitate the human understanding of dynamic networks, but the complexity and volume of the network dynamics poses a challenge to research. Today’s telecommunication networks are huge, highly dynamic and heterogeneous, social media channels can have hundreds of thousands of messages per second, and microsecond trading at financial markets creates millions of data points per second. The development of sensor networks e.g. for electric grids and smart homes is advancing fast, and will lead to huge amounts of network data that need to be analyzed. Recently, approaches to combine multiple sources of structured and unstructured data have become more and more popular, e.g. to support decision making and fraud detection at the capital markets by combining financial data with sentiment analysis of social media data. New tools for analysis that are capable of handling large dynamic networks are required to support decision makers, e.g. providers, regulators, and policy makers. Limits apply to all steps of the visual analytics pipeline, including the analysis, modeling, visualiza- tion, and interactive exploration of the data. These limits are due to computational limitations and lack of appropriate methods, but also the limitations of the human capability to perceive and process visual information. For large scale dynamic data, just stepping through discrete time steps is not an option, a visual analytics system has to analyze and reduce or aggregate data and time information for visual exploration first, and then has to give guidance to the user to find relevant time points and changes, without e.g. levelling out interesting patterns. Analysis methods have to work in an incremental fashion, and noise and uncertainty in the data might further complicate the processing. For example, while the resolution of brain imaging methods increased largely over recent years, the granularity might still be too coarse to capture the finer patterns in brain activity. The development of solutions that are successful in practice thus requires combined efforts in network analysis and visualization, and the development of appropriate interactive interfaces that allow to be aware and to overcome shortcomings in the used technology. Several research directions are concerned with the development of methods to support the analysis of dynamic network data, including information and data visualisation, data analytics, machine learning, and human computer interaction. The collection, processing, and visualization of complex network data for analysis purposes presents a huge challenges for all of those areas. While visualization of changes is an important task, interfaces that allow the user to intuitively interact with the visualization in an efficient manner are a further challenge. An important aspect is to tailor the representation towards the actual use-case. In a similar way, network analysis methods need to be adapted to online streaming scenarios and the potentially huge data volumes. Knowledge discovery methods need to cope with large amounts of streaming data, but also with potential uncertainty and incompleteness of processed data. Patterns in the data that show either outliers of particular interest, e.g. for fraud detection in financial data or functional subnetworks in brain activity, need to be detected and classified. For some application areas and use-case it is possible to come up with classes of typical or interesting changes that then can be used during automated analysis and aggregation. For other applications, it might be required that a system is able to adapt to the changes in the data, and learns from it as well as from the user feedback. Overall, dynamic network visual analytics poses a research challenge not only due to the dynamic nature of the data, but also its volume and complexity.
2. Topics and Aim of the Seminar
The development of efficient methods for dynamic network visual analytics requires a multi-disciplinary approach that spans not only the classical disciplines related to data visualisation and interaction, visual analytics, information visualisation, and graph drawing, but also includes data sciences and network analysis. While researchers in all these fields tackle the problem of dynamic network analysis, we feel that a concerted effort will be best suited to advance the current state and will lead to new fruitful collaborations. The seminar has the aim to bring together experts in the listed fields and people from application areas to first have an exchange across the fields on the state of the art and current challenges, and then to state and tackle the most pressing research questions. We want to discuss dynamic network visual analytics starting with a few core real world examples, where practitioners present the setting and challenges and discuss requirements and constraints for potential solutions with visualization and analysis experts. To this end, we will select two or three practitioners to prepare presentations of real world problems. In order to facilitate a concerted effort of different research fields, a main topic will be the efficient combination of solutions for the different tasks in a visual analytics approach for dynamic networks. Subtopics that focus on particular aspects will be discussed and evaluated together such that there is an awareness for the limits and potentials of the respective solutions. These topics include network analysis and aggregation to efficiently capture changes in dynamic networks and to detect outliers, patterns and trends, graph layout, data and time-series visualization and interactive exploration. Our aims for the seminar are as follows:
- Bring together researchers from several communities that can contribute to dynamic network visual analytics research, including Visual Analytics, Information Visualization, Graph Drawing, Network Analysis, and researchers and practitioners from application
- Identify core challenges based on both ongoing research and real-world examples, g. regarding computational efficiency, visual complexity, and interaction.
- Examine where existing approaches can be extended and where completely new methods need to be developed to cope with the recent huge rise in complexity, velocity and volume of dynamic network data for network analysis and interactive visualization, e.g. through collaborative
- Investigate the embedding of dynamic network data and visualizations into the interfaces and visualizations commonly used in practice today, e.g. combining trading networks with analysts communications or brain networks with imagery.
- Investigate how we can adapt existing visual analytics techniques for dynamic graphs to interact with and be executed on a cloud computing framework.
- Investigate the use of alternative representations than node-link visualizations for dynamic net- works, e.g. matrices or splatting maps, and the combination of multiple representations.
- Study efficient scalable approaches to detect and visualize repetitive behavior, e.g. recurring pat- terns. In particular, we will explore how statistical analysis approaches and pattern recognition techniques can be used to detect repetitive behaviors in rapidly evolving networks.
- To define and document research challenges for the future.