NO.072 Analytics on complex networks: scalable solutions for empirical questions
February 8 - 11, 2016 (Check-in: February 7, 2016 )
Organizers
- George Fletcher
- Eindhoven University of Technology, The Netherlands
- Taro Takaguchi
- National Institute of Informatics, Japan
- Yuichi Yoshida
-
National Institute of Informatics, Japan
-
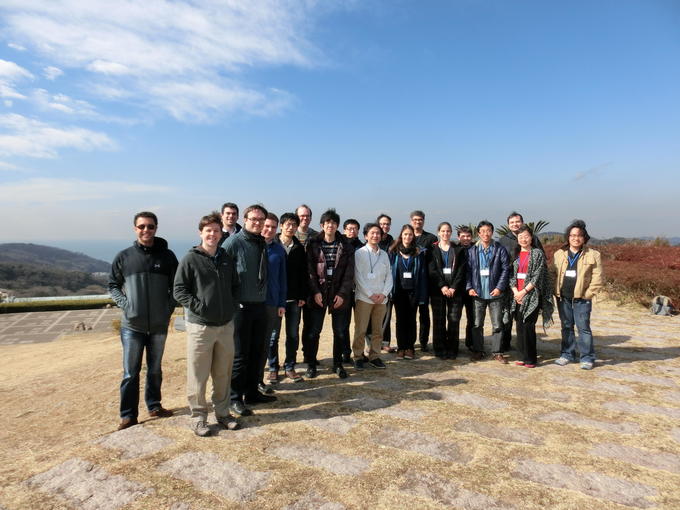
Overview
Real-world networks, such as web graphs, social networks, and biological networks, have remarkable topological features in common: the scale-free property, that is, the degree distribution obeys a power-law function, and the small-world property, that is, the average distance between two vertices is small. These networks are collectively referred to as complex networks and have been intensively studied in the last decade.
In their empirical study of complex networks, researchers across the fields of data engineering, theoretical computer science, and network science are developing solutions for analytics. Due to rapid growth of the sizes of real-world networks, however, we are facing challenges in scaling these tools, as we will describe below. We desire to solve these issues by bridging the knowledge in the three fields with the single key notion: complex networks.
Researchers in the engineering of data-intensive systems, such as database and data mining systems, have traditionally focused on the study of scalable solutions for analytics on big data collections. With the increasing availability and broad interest in massive complex networks, researchers in data engineering have hence focused considerable effort in recent years on scalable mining and querying over such data collections. However, very little work has been done on coordinating the results of these investigations with the foundational work in this area in the theoretical computer science and network science communities. Stronger ties between these communities would help deepen the understanding and development of theoretically grounded analytics solutions for the empirical investigations of interest in the broader scientific community.
From the perspective of theoretical computer science, the important point is that we need algorithms for real-world networks but not for all the possible networks. The majority of theoretical studies on graph problems pay attention to worst-case time complexity or average-case time complexity, which may have little to do with real-world networks. On the other hand, practical algorithms on graph problems proposed in the fields of databases, data mining, and machine learning have shown their efficacy on real-world networks, but these algorithms lack theoretical groundings to understand why they work well in practice. By applying theoretical analysis frameworks to these algorithms, we want to answer foundational questions such as “what is the right time complexity of this particular problem when the given graph is a complex network?” and “why does this particular heuristic make the algorithm faster or more accurate when the given graph is a complex network?”
Network science, a new subfield of statistical physics seeking universality hidden behind real-world networks, can move on to a new stage with aids provided by data engineering and theoretical computer science. For example, a large number of models describing growth processes of complex networks and information spreading on them have been studied in network science. However, their computational properties are largely unexplored, which prevents us from discussing their properties with rigorous theoretical groundings. As another example, various kinds of vertex centralities, which measure the importance of vertices, have been proposed and applied to network data. However, the majority of these centralities are computationally hard and efficient (approximation) algorithms should be studied to apply them to real-world networks at a large scale. In theoretical computer science, strong analytical tools for graphs such as graph minor theory and spectral graph theory have been developed and these theories may shed new light on novel characteristics of complex networks.
As we have seen, complex networks are studied in diverse fields from different perspectives. The main goal of this workshop is bringing researchers in these fields together to take the first steps towards bridging works on scalable analytics over complex networks.