NO.061 Dimensionality and Scalability II: Hands-On Intrinsic Dimensionality
June 29 - July 2, 2015 (Check-in: June 28, 2015 )
Organizers
- Laurent Amsaleg
- CNRS- IRISA, France
- Michael E Houle
- Natinal institute of informatics, Japan
- Vincent Oria
- New Jersey Institute of Technology, USA
- Arthur Zimek
- Ludwig-Maximilians-Universität München, Germany
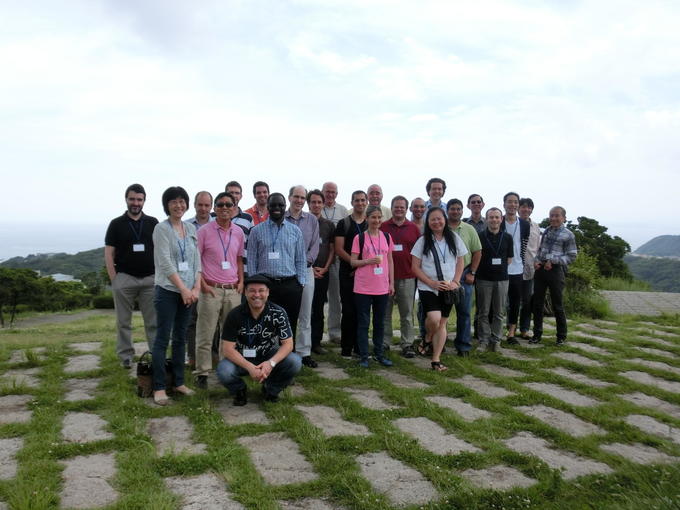
Overview
Description of the Meeting
Background
For many fundamental operations in the areas of search and retrieval, data mining, machine learning, multimedia, recommendation systems, and bioinformatics, the efficiency and effectiveness of implementations depends crucially on the interplay between measures of data similarity and the features by which data objects are represented.
When the number of features (the data dimensionality) is high, similarity values tend to concentrate strongly about their means, a phenomenon commonly referred to as the curse of dimensionality. As the dimensionality increases, the discriminative ability of similarity measures diminishes to the point where methods that depend on them lose their effectiveness. The effects of the curse of dimensionality on search and clustering methods are well-known and well-documented. Domain transformation strategies such as dimensional reduction and feature selection can improve performance to some extent, but the fundamental difficulties associated with high dimensionality nevertheless persist.
Over the past decade or so, new characterizations of data sets have been proposed for assessing the performance of particular methods. Such characterizations include estimations of distribution, estimation of local subspace dimension, and measures of intrinsic dimensionality of data. Although the applications affected by the curse of dimensionality vary widely across research disciplines, the characterizations and models of data that can be applied to analyze the performance of solutions are very general. Across the different disciplines, the data models and data characterizations that have been proposed are quite similar. Unfortunately, researchers from one domain are typically unaware of what researchers from other domains have developed.
NII Shonan Meeting on Dimensionality and Scalability (May 2013)
In May 2013, a NII Shonan Meeting was held to bring together researchers and students active in the areas of databases, data mining, pattern recognition, machine learning, statistics, multimedia, bioioinformatics, visualization, and algorithmics who are currently searching for effective and scalable solutions to problems affected by the curse of dimensionality. The main objectives of this workshop were to survey the existing approaches used in dealing with the curse of dimensionality in these various disciplines, to identify their commonalities, strengths and limitations, and to clarify the potential impact of such approaches on core tasks such as search, classification, and clustering.
During the four days of the Meeting, 19 participants participated in brainstorming sessions, identifying future directions for research on dimensionality and scalability. 10 survey presentations were made on the impact of dimensionality in the disciplines of databases, data mining, multimedia and machine learning. Small working groups eventually focused on the interplay between intrinsic dimensionality and topics in such areas and topics as clustering and outlier detection, multimedia, graphs and networks, and feature selection. The discussions quickly focused on the need to develop and exploit practical estimators of intrinsic dimensionality.
See the report on this meeting under /docs/No.2013-4.pdf
Day Seminar on Dimensionality and Scalability (March 2014)
In the months after the Shonan Meeting, participants continued to investigate the theory and applications of intrinsic dimensionality. Several key outcomes were presented at a special one-day seminar organized in March 2014 at NII, which was attended by 8 of the participants from the first Shonan Meeting.
On the theoretical side, the use of Extreme Value Theory enabled the development of several practical estimators of a newly proposed model of the intrinsic dimensionality of distance distributions. In parallel, several contributions allowed for a better understanding the impact of intrinsic dimensionality of data sets on the quality of similarity search and supporting indexing techniques. Scoring functions based on intrinsic dimensionality were also presented for the detection of outliers. In addition, a method was presented by which k-nearest-neighbor graphs construction could be combined with simultaneous local dimensional reduction (data sparsification). The normalization of scores and distances for ensemble methods was proposed as a means of compensating for the adverse effects of high dimensionality.
NII Shonan Meeting on Dimensionality and Scalability II: Hands-On Intrinsic Dimensionality (May 2015)
We now propose a second NII Shonan Meeting on Dimensionality and Scalability, in order to (i) disseminate more widely the results obtained since 2013; (ii) leverage on the initial collaborations to consolidate research agendas; and (iii) obtain feedback from new researchers concerned with the general problems of dimensionality and scalability.
The detailed objectives of the meeting are:
1. Sharing our latest discoveries, so as to increase the visibility of our contributions among the community that has arisen since the meeting in 2013. With the new model of intrinsic dimensionality of continuous distance distributions, our theoretical perspective is now better established.
We now have estimators that can compute local values of intrinsic dimensionality, and procedures for making use of these estimators to prune search spaces, filter noisy points, and enhance indexing and clustering. It is crucial to disseminate this knowledge so that progress can continue to be made on as many fronts as possible, and to find other use-cases where such estimators can help in alleviating the effects of the curse of dimensionality.
2. To foster collaborations among this new community of researchers. The first Shonan workshop fully succeeded in bootstrapping collaborations that resulted in the contributions presented during the one-day seminar in March 2014. Four major collaboration topics have emerged: deeper investigation of the complex relationships between intrinsic dimensionality and high-dimensional indexing and clustering; clarification of the relationship between shared nearest neighbors, hubness and intrinsic dimensionality; the characterization and improvement of outlier detection using intrinsic dimensional estimators; and gaining an understanding of the properties of distance ensembles in terms of their constituent similarity measures.
One goal of the second edition of the Shonan workshop is to both consolidate these existing collaborations and to initiate new ones.
3. The third goal is to bring together new potential collaborators. Since the first Meeting, we as a community have gained a great maturity on the theoretical side as well as on the practical side. It will be obviously beneficial to present these more evolved ideas to those researchers concerned with some aspect of dimensionality and scalability who could not attend the first Meeting.