NO.128 Workshop on Patient Similitude: Combining Histopathological Images & Multiple-Scale Molecular Phenotypes
November 12 - 15, 2018 (Check-in: November 11, 2018 )
Organizers
- Raghu Machiraju
- The Ohio State University, USA
- Jens Rittscher
- University of Oxford, UK
- Motonori Ota
- Nagoya University, Japan
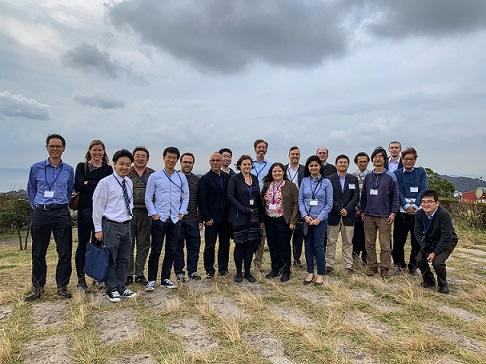
Overview
Description of the meeting
Description of the Workshop Patient data is increasingly available in many forms including both genomic and various phenotypic forms including – transcriptomic, epigenetic, proteomic, histopathological, radiological, and clinical variables. Repositories such as The Cancer Genome Atlas (TCGA) contain a multitude of patient records which can be used for patient stratification, disease subtype/biomarker discoveries, all essential elements in the era of precision medicine. While there is the promise that the integration of all this available information will help to realize a more personalized and precise treatment, salient fundamental and foundational questions need to be addressed before this vision can become a reality. For instance, two patients afflicted with a Stage 3 manifestation of a certain cancer can report a similar gene panel yet the ensuing analysis can still lead to different survival regimens since the expressed regulatory microRNA expression are different. The outcome to treatment and hence the overall response is indeed very different.
Goals of the Workshop: In this workshop we address the methodological and application specific challenges of a principled data integration in the genomic and phenotypic space in a highly structured and systematic way. As histopathology plays a significant role in clinical decision making and patient management, it serves as the lynchpin of integrative methods. We aim to promote approaches that build on the existing diagnostic workflows but extend these to integrate the other available information. This workshop provides an unique interdisciplinary setting to bring together clinical scientists, experts in bioinformatics along with computer scientists and biomedical engineers to discuss these challenges and nucleate a scientific community.
Participation: All invitees will be asked to submit a 1-page response to questions on the formulation of the problem and likely methodologies of integrated omics. These 1-page responses will be used to organize the workshop with discussions being led by specific
senior members. The program will reflect the entire scale spanning the genome and its various phenotypic forms and will allow an opportunity to examine the rubrics for an integrated way to compare patients and models. We will also encourage participants to provide hands-on demonstrations of relevant software tools and curated data sets. Lastly, we will discuss what requirements these tools need to fulfill to be of value to the emergent research community. This workshop will provide the catalyst for new international collaborations. Edited proceedings in the form of communications will also be forthcoming.
Workshop format To reiterate, a salient problem is one that will determine the similarity between patients in the multi omics context. More specifically, how does one compute the composite edge weight (or distance) between two nodes of a graph in this context ? And other related questions in this context. In order to address these questions we will bring together leading clinical scientists, image analysis and machine learning experts as well as bioinformaticians who will cover the following areas of expertise:
- Computational pathology – How can recent developments in computer vision and machine learning be leveraged to represent tissue architecture context and reflect the intrinsic continuous nature of disease progression?
- Molecular Diagnostics – Novel approaches for imaging molecular and genetic markers in the tissue architectural context? Or delineate successful correlative and modeling methods that have predictive and prognostic abilities.
- Systems pathology – How can we make the transition from today’s histology-centric and categorical disease descriptions to integrated representations that effectively support precision medicine?